Nicholas Waytowich
Brain-Computer Interface and Neural-signal Processing Researcher
Spatial Decoupling of Targets and Flashing Stimuli for Visual Brain-Computer Interfaces. [PDF]
N. Waytowich, D. Krusienski
Published, Journal of Neural Engineering
Problem: Code-Modulated Visual Evoked Potentials (c-VEPs) have been proven to achieve among the highest information transfer rates for non-invasive BCIs. However, one issue with current c-VEP paradigms and visual-evoked paradigms in general, is that they require direct visual fixation of flashing stimuli. Current c-VEP interfaces are often visually unpleasant and can be irritating and fatiguing to the user.
Solution: A novel c-VEP BCIU paradigm is presented that attempts to perform spatial decoupling of the targets and flashing stimuli using two distinct concepts: spatial separation and boundary positioning. For the paradigm, the flashing stimuli form a ring that encompasses the intended non-flashing targets, which are spatially separated from the stimuli. The user fixates on the desired target, which is classified using the changes to the EEG induced by the flashing stimuli located in the non-foveal visual field. Additionally, a subset of targets is also positioned near the stimulus boundaries, which decouples targets from direct association with a single stimulus.
Results: Results from 11 subjects showed offline classification accuracies of targets with non-foveal stimulation are comparable with the traditional direct-foveal stimulation targets. Online results from 5 subjects confirmed the offline results with an average accuracy across subjects of 92.7% for a 4-target condition. The offline analysis also indicated that targets positioned at or near the boundaries of two stimuli could be classified with the same accuracy as traditional superimposed (non-boundary) targets.
Significance: This study shows that c-VEPs can be detected and accurately classified to achieve comparable BCI performance without requiring potentially irritating direct foveation of the flashing stimuli. Furthermore, this study shows that it is possible to increase the number of targets beyond the number of stimuli without degrading performance.
Current Research
-
Brain-Computer Interfaces.
-
Multivariate neural-signal Processing.
-
Machine Learning.
-
Experimental Design.

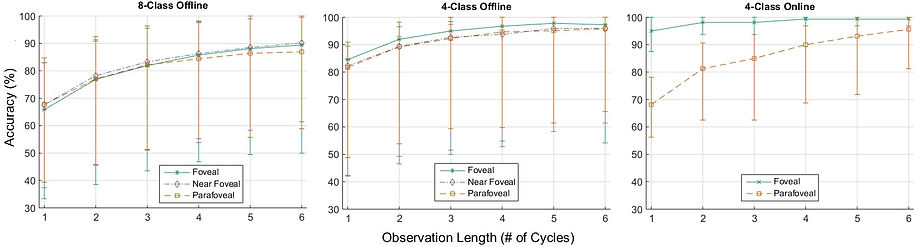

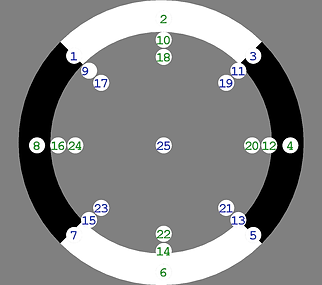
Novel Characterization of the Steady State Visual Evoked Potential Spectrum of EEG. [PDF]
N. Waytowich, D. Krusienski
BrainKDD: International Workshop on Data Mining for Brain Science, 2014
Problem: Steady-State Visual Evoked Potentials (SSVEPs) are oscillations of the electroencephalogram (EEG) observed over the occipital area that exhibit a frequency corresponding to a repetitively flashing visual stimulus. SSVEPs have proven to be very consistent signals for rapid EEG-based brain-computer interface (BCI) control. However, due in part to perceptual and neurophysiological aspects, SSVEP signal detection biases exist for dierent stimulation frequencies. Furthermore, these biases tend to differ across subjects.
Objective: In this work, in order to provide a better characterization of the SSVEP spectrum for BCI applications, 22 subjects were stimulated with an LED array that flashed according to a chirp signal having a frequency that varied over the typical functional range of SSVEP from 5.5-34.5 Hz. The resulting EEG was analyzed using Canonical Correlation Analysis (CCA) to elucidate the stimulus frequencies that produce the best discriminability for practical use.
Results:Subjects achieved an average accuracy of 72.2% using a six-class paradigm with a standardized set of stimulus frequencies. However, whenusing a subject-specic frequency set (i.e. frequencies optimized for each subject), the average accuracy significantly increased to 83.7% (p = 0:03).
Significance: The results show that inherent SSVEP response dierences exist between subjects, which can have a signicant effect on performance. This approach also establishes a framework for a rapid optimization of subject-specific frequency profiles.

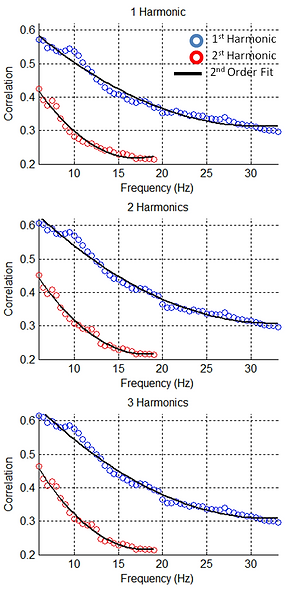

Development of a Flexible software platform for VEP based BCIs.
N. Waytowich
Flexible software is rarely found in the context of BCI systems. Most BCIs today are implemented in the laboratory strictly for use in research studies. As such, the underlying software that controls the visual stimulation of a BI is fixed to the parameters of the associated study. This rigid system model is not conducive for practical BCIs. SSVEP stimuli that are individually tailored to the individual can greatly increase the detection accuracy and robustness of the SSVEP-BCI. A software platform is developed with configurable, on-the-fly parameters that allow for real-time tailoring of stimuli. This flexibility allows the user to preferentially select stimuli that they find the most visually appealing by adjusting parameters such as stimulus frequency, color, size and position. This aids in the reduction of visual fatigue and increases overall system comfort. The software platform (example screenshots shown below), was written in C# and is capable of displaying visual stimuli of any type for a VEP BCI. The current version of the software contains multiple embedded applications for various BCI tasks such as wheelchair control (spatial navigation), neruofeedback, google maps control, virtual navigation, as well as several BCI related games. The software is extensible so that more applications can be added in the future.
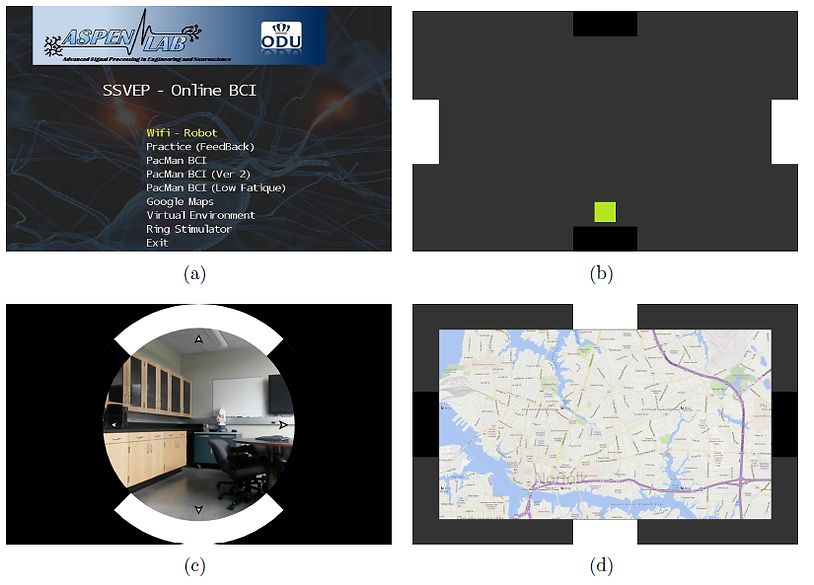
Part (a) shows a screenshot of the home-screen for the proposed software framework. Part (b) shows a standard 4-target SSVEP system with a green bar as feedback. Part (c) shows the ring paradigm implemented with SSVEP to control a mobile web-cam robot in a spatial navigation task. Part (d) shows a 4-target SSVEP system to control Google maps.
Multiclass Steady-State Visual Evoked Potential Frequency Evaluation using Chirp-Modulated Stimuli. [PDF]
N. Waytowich, D. Krusienski
In Submission, Jan 2015
Problem: Steady-State Visual Evoked Potentials (SSVEPs) exhibit among the most accurate and robust EEG-based brain-computer interface (BCI) control. However, due in part to perceptual and neurophysiological aspects, SSVEP signal detection biases exist for different stimulation frequencies. Additionally, there is no standardized set of SSVEP stimulus frequencies or frequency selection method, thus, many studies select SSVEP stimulation frequencies somewhat arbitrarily.
Objective: In this work, 17 subjects were stimulated with an LED array that flashed according to a chirp-modulated signal having a frequency that varied linearly over the typical functional range of SSVEP. The resulting EEG was analyzed using canonical correlation analysis (CCA) and a genetic algorithm (GA) was implemented to determine generalized stimulation frequency sets over a continuum of simulated multiclass BCI classification scenarios.
Results: The results show that distinct frequency feature groupings exist over the different multiclass scenarios, and that these groupings result in different information transfer rates.
Significance: These offline results provide a comprehensive evaluation of the most discriminable frequency feature sets for a given number of classes, which should serve as a reference and a guide for standardization of generalized SSVEP stimulus
frequencies.
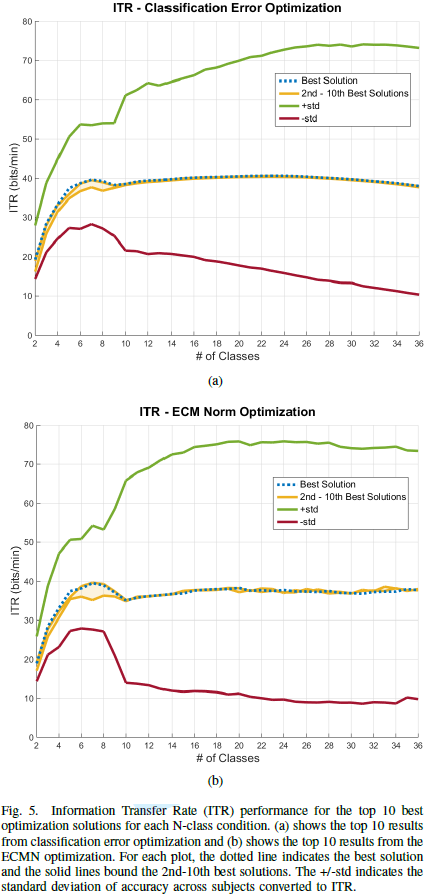
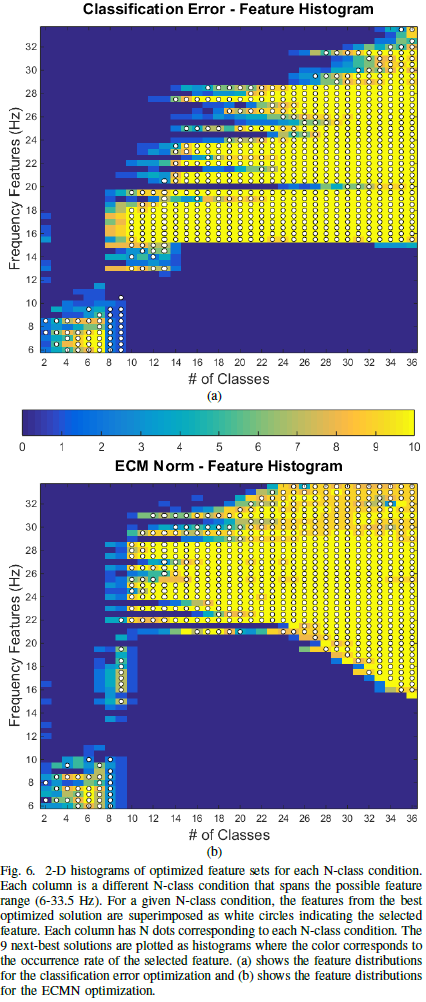